
NVIDIA RTX 4090: The Game-Changer for Deep Learning and AI
Learn why the NVIDIA RTX 4090 is a powerful choice for AI and deep learning, offering top-tier performance and cost-effective solutions for researchers and developers.
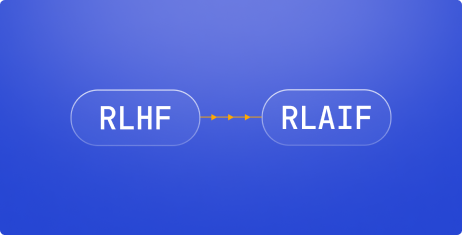
The NVIDIA RTX 4090, initially released for the PC gaming market in October 2022, has quickly established itself as a formidable contender in the realm of deep learning, artificial intelligence, and scientific computing. This article explores why the RTX 4090 is an excellent choice for data scientists, AI researchers, and developers looking to elevate their deep learning projects.
Architecture and Specifications
At the heart of the NVIDIA 4090 lies NVIDIA's cutting-edge Ada Lovelace architecture. This GPU boasts impressive specifications that make it a true powerhouse for deep learning tasks:
- 16,384 CUDA cores
- 512 4th generation Tensor Cores
- 24 GB of GDDR6X VRAM
- 2.23 GHz boost clock
- 1 TB/s memory bandwidth
- PCIe 4.0 interface
These specifications translate to exceptional computing power, enabling the RTX 4090 to tackle complex deep learning tasks such as facial recognition, natural language processing, and computer vision with ease.
Performance Comparison
When compared to its predecessor, the RTX 3090, the NVIDIA 4090 shows significant improvements:
- TF32 training throughput is 1.3x to 1.9x higher than RTX 3090
- FP16 training throughput is 1.3x to 1.8x higher than RTX 3090
- Training throughput per dollar is 1.2x to 1.6x better than RTX 3090
- Training throughput per watt is comparable to RTX 3090, ranging from 0.92x to 1.5x
These improvements make the RTX 4090 an attractive option for those looking to upgrade their deep learning setup.
CUDA Library Support and AI Acceleration
The RTX 4090 supports the latest version of NVIDIA's CUDA-X AI Library, providing data scientists and developers with a rich set of optimized algorithms for deep learning applications. Key features include:
- Compatibility with popular AI libraries such as TensorFlow and PyTorch
- Support for CUDA-optimized libraries like cuDNN, TensorRT, and CUDA-X AI
- DLSS AI upscaling, which can improve the performance of deep learning models by up to 200%
These features allow for quick implementation of GPU acceleration in projects and reduce development time for high-performance computing applications.
Multi-GPU Scaling
While the RTX 4090 doesn't support NVLink, multi-GPU setups using PCIe 4.0 still show promising results:
- Most models achieve close to 2x training throughput with two GPUs
- Some models, like BERT_base fine-tuning, show sub-optimal scaling (around 1.7x throughput for 2 GPUs)
- 2x RTX 4090 consistently outperforms 2x RTX 3090 (with NVLink) across various deep learning models
Cost-Effectiveness for Deep Learning
The RTX 4090, priced at $1599, offers excellent value for those interested in deep learning, especially when compared to its predecessors and professional-grade GPUs:
- Faster training throughput than the previous flagship, the RTX 3090
- More cost-effective performance for deep learning tasks
- An affordable option for students, researchers, and creators on a budget
Accessibility through GPU Rental Services
While the RTX 4090's performance is impressive, the upfront cost of $1599 may be prohibitive for some researchers, students, or small businesses. Fortunately, GPU rental services have emerged as a cost-effective solution to access this cutting-edge technology.
At PoolCompute, we're offering RTX 4090 rentals starting at just $0.83 per hour. This makes it easier than ever for you to access top-tier GPU performance for your deep learning and AI projects without breaking the bank. You can easily get started through our marketplace.
Renting GPUs offers several advantages:
- Cost-effectiveness: Pay only for the time you use, avoiding large upfront investments.
- Flexibility: Scale your resources up or down based on project needs.
- Access to latest hardware: Use the most recent GPU technology without worrying about hardware becoming outdated.
- No maintenance overhead: Avoid issues related to hardware upkeep and cooling.
If you're a researcher, student, or business aiming to tap into the power of the RTX 4090 for deep learning, our GPU rental service at PoolCompute offers a flexible and budget-friendly solution. With us, you can access this high-performance hardware without the need for a big upfront investment, making it easier to tackle your most demanding AI projects.
Considerations and Limitations
While the RTX 4090 is an excellent choice for deep learning, there are some factors to consider:
- Size: At 61 mm (2.4 inches) width, it requires 3.5 PCIe slots
- Power consumption: 450W TDP, requiring a minimum 850W power supply
- Lack of NVLink support for multi-GPU setups
- Not designed specifically for data center use (consider professional GPUs like the A6000 for such applications)
Is There a 4090 Ti?
As of now, NVIDIA has not released a 4090 Ti model. The RTX 4090 remains the flagship consumer GPU in the current generation. However, rumors and speculation about a potential 4090 Ti continue to circulate in the tech community.
Conclusion
The NVIDIA RTX 4090 represents a significant leap forward in GPU technology for deep learning applications. Its powerful Ada Lovelace architecture, coupled with an impressive array of CUDA cores and Tensor cores, makes it capable of handling the most demanding AI and machine learning workloads.
For data scientists, AI researchers, and developers looking to push the boundaries of what's possible in deep learning, the RTX 4090 offers a compelling combination of raw power, advanced features, and cost-effectiveness.
As deep learning continues to evolve and tackle increasingly complex challenges, having access to GPUs like the NVIDIA 4090 can make all the difference in staying at the forefront of innovation in this exciting field. Whether you choose to invest in your own hardware or leverage rental services, the RTX 4090 stands ready to accelerate your deep learning projects to new heights.
Decentralized computing for AGI.
Decentralized computing unlocks AGI potential by leveraging underutilized GPU resources for scalable, cost-effective, and accessible research.